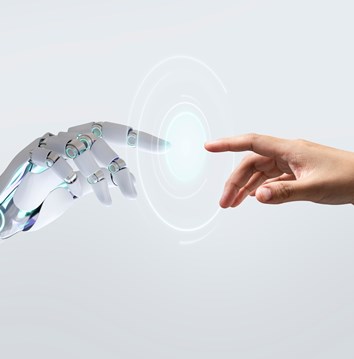
Creating competitive advantage with generative AI for mid-market companies: Learnings and pitfalls
While the benefits of generative AI are becoming clear, precisely how best to harness its power to drive real value and growth is less so. Inflexion invited its portfolio to learn from Humanloop about going beyond ChatGPT to build solutions delivering competitive advantage.
Organisations of all sizes are waking up to the realm of possibilities offered by the rapid development of generative AI, including OpenAI's ChatGPT and GPT-4. Traditional AI required substantial human resource to deliver meaningful results, and so didn’t gain much traction for firms that weren’t tech enabled. But the latest offerings differ and promise to be a real game-changer.
Off-the-shelf tools such as Github Copilot or Jasper may increase developer productivity or help marketing, but they are rapidly becoming commodities which generate little competitive advantage. “We see a lot of potential in automating customer interactions and co-piloting workflows in the back-office,” says Jan Beitner, Assistant Director for Data & AI at Inflexion. Creating value does not require full automation, but you can start co-piloting, i.e. let generative AI suggest outcomes and drafts to humans.
A recent event hosted by Inflexion examined the best approach towards AI whilst highlighting a few common pitfalls.
Assembling the right team
Assembling the right team for your generative AI projects is critical. Most important, business input is indispensable for ensuring that your AI solutions align with your company's goals and challenges. In fact, business stakeholders can and should meaningfully contribute to prompt engineering, i.e. asking the right questions to AI in natural language.
Because large language models (LLMs) are so complicated to operate, they are available off-the-shelf from the large cloud providers. “This means data scientists are optional for a generative AI project, while engineers are necessary for technical implementation and integration into your systems,” Jan explains. “Often full-stack and front-end engineers should get involved, particularly because many applications involve building websites and they are used to taking the end-users perspective.”
Moving fast
Building a proof-of-concept (PoC) can be very fast and achieved in a matter of days. Even going to production can be done in a matter of weeks rather than months because the complexity of running generative AI solutions is handled by cloud providers, allowing you to focus on solving the business problem.
Focus on quality over cost
While it may be tempting to fine-tune your AI models from the outset, it's essential to avoid premature optimisation. “Start with GPT-4, the most powerful model currently available, and focus on getting your application working. You can always fine-tune or switch to a more cost-effective solution later,” says Raza Habib, CEO of LLM application platform Humanloop.
Integrating your own data is often better achieved with retrieval-augmented generation (RAG), a technique that adds potentially useful snippets of your own data as part of the question to the LLM. It requires no fine-tuning, hence requires less technical expertise, and as a bonus also leads to fewer hallucinations, i.e. keeping the LLM output factual.
Beware underestimating the power of fine-tuning at a later stage. Raza adds “With just a few hundred examples, you can achieve excellent results while massively saving on costs and improving generation speed and thus customer experience.”
The importance of systematic evaluation
To ensure the success of your generative AI projects, a clear and systematic evaluation process is crucial. “This is different from traditional software development in that designing generative AI prompts is more an art than pure engineering, so understanding the quality of your solution will be often more difficult and require careful evaluation of all components of the application,” Raza says. And to avoid optimising for the wrong outcomes, Jan suggests the process should involve strong collaboration between business and technical stakeholders.
There are three primary types of evaluation to consider:
- Implicit Evaluation: Monitor the usage and acceptance of your AI-generated output by users. For example, GitHub Copilot examines how much of its suggested code remains in the user's codebase over time, while ChatGPT checks if users copy its output.
- Explicit Evaluation: Encourage users to provide feedback, such as thumbs up or down, on the AI-generated content. Invest time in designing a feedback mechanism to capture valuable information from the beginning.
- Automatic Evaluation: Leverage LLMs to judge the quality of AI-generated output. This approach also helps the output to be factually correct, i.e. further reduce hallucinations Further, it prevents toxic outputs that could damage companies’ reputations.
Choosing the right tools
Generative AI specific tooling includes vector databases such as Pinecone or OpenSearch for storing your own and user data, and an evaluation tool such as Humanloop. “Traditional business intelligence tools do not work well with text, images, or video data, which are common in- and outputs of generative AI,” Raza points out. The technology landscape for generative AI is still evolving, with no clear winners in terms of tools and platforms and, so far, limited differentiation of vendors. “The best tech available on the modelling side is GPT-4 by OpenAI,” Jan concludes.
Chambers And Partners
Chambers is an independent research, data and analytics company that evaluates and ranks law firms and individual lawyers based on their expertise, client service, and market reputation. Since Inflexion’s investment, the company has transformed from a print publisher into a digital powerhouse through a comprehensive transformation programme.
Insight reports are a core Chambers’ product which provide a unique insight into law firms performance through Client and Market Intelligence, and pinpoint areas of improvement for law firms to focus on. A fifth of the time required to create these reports is spent on quote cleaning, which involves reviewing interviews with lawyers, putting their statements into grammatically correct English, and removing sensitive, personal information. It is crucial to stay as close as possible to the original quote without changing its meaning.
Quote cleaning was identified as an ideal candidate for generative AI-powered automation. In just a few weeks from the initial inception of the idea, a prototype went into production, with a ChatGPT-cleaned quote proposed to research analysts who determine whether to iterate further. With this first rough version, around 10% of quotes did not require research analyst input and there is an opportunity for up to 40% to be AI generated with only minor changes.
“We were only able to do this because Chambers’ processes were already digitised, so we could integrate generative AI efficiently, and evaluate its impact,” says Tim Noble, CEO of Chambers.
Contact